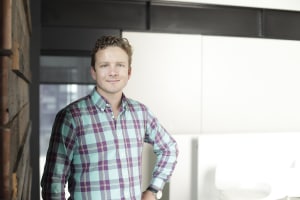
As consumers of content, information and products, we are in a constant state of data transmission. Our location, interests, friendship network and personal details via login, transaction or loyalty systems are constantly accessible. As marketers who draw on this data for advertising there are many opportunities to delight or distain. A positively received use of data may be relevant content suggestions or hotel deals for a destination you have just booked flights to. It is much easier in this case to delight as the advertising is highly relevant to a person’s behaviour.
Over the past 18 months our industry has placed a heightened focus on quality assurance for digital inventory (ad impressions). Is your ad deemed to be viewable by an independent third party? Is it a human or robot? Is it appearing on the website you think you are buying? All have made a valuable contribution to marketing effectiveness. This year we need to build on this by increasing our focus on quality assurance for paid data. To date there is no standardised measure for data quality. Scale across devices, accuracy and efficacy are of course the most desirable measures of success, but if you dig deeper and start to ask questions, it can be much more difficult to define what quality data is.
The first question to ask relates to the data’s provenance. Where it has been sourced from and what types of data are being used. Furthermore, is the targeted audience based on verified or inferred methodology? If verified, is it claimed (survey), declared (membership/login/Census) or actual (transactional data)? Each are very different data sets that require robust analysis and hygiene. Having a data strategy that is grounded in reality, not dreams, desires or misinformation is key to getting this right.
Another consideration should be how different types of paid data products are constructed. In the world of targeted advertising, there are generally two methods of construction.
The first is data that comes from a single source. An example of this is transaction, loyalty, login or credit applications. The owners of these datasets have a consistent ‘deterministic’ data set which is easier to verify or keep updated. It can also be matched to other data sets, such as email members for example. Herein ‘second party’ datasets can be created. An example of this is how Yahoo7 matches anonymised email member data to supermarket loyalty and transactional databases with Quantium. This type of data has the potential to be highly accurate, and is often termed people based marketing.
Being able to communicate to consumers with relevant messaging is a compelling opportunity for marketers. However, scale can be an issue depending on your segment size, number of ad units available, and inventory requirements. Thus the need for scale and a second variety of paid data, which is where ‘Third party’ data comes in.
Third party data is often constructed from multiple sources. Data signals are collected, categorised and aggregated into an audience segmentation model. Examples of data signals include website interactions, mobile device location, online surveys or ad clicks. Recency and frequency of one’s browsing behaviour is the most common method of qualifying intent to purchase. It can however be difficult to assess the data’s provenance. More often than not, the third party will not disclose the contents of its warehouse. Many raw producers are monetising customer data as an incremental revenue stream. If this revenue stream is disclosed there is a risk their traditional advertising sales revenue will be cannibalised. This sharing of data with ‘third parties’ or ‘affiliates’ must always be disclosed on the producers website or during the signup process but it often does not go much deeper than that.
This of course is about to change with the introduction of GDPR, and the requirement for greater transparency on the commercial use of personal data. There are also changes underway in the data monetisation space as data owners seek to gain more control over how their data is used, and the price that is paid for it. Software is now able to facilitate one-to-one data sharing and trading between two entities. The data owner is able to control who accesses their data, and what attributes are shared for an agreed price. For the more sophisticated data owner this is a real alternative to the warehouse solution provided by third party aggregators.
It really does pay to question where your paid data comes from and how robust its segmentation methodology is. While efficacy is important, it is beneficial to dig deeper and start from an informed position. As we seek to ‘humanise’ the data we must not forget to actually deliver on that promise. Establishing internal benchmarks on data quality and putting in place a culture of rigour, trust and respect for the consumer is both essential and our responsibility.
Yahoo7 head of data and targeting Dan Richardson